Artificial Intelligence (AI) has revolutionized various aspects of human life from enhancing industrial efficiency with Industry 4.0 and smart IoT devices to improving healthcare with image processing and machine learning in recent years. While most AI advancements are led by first-world countries some underdeveloped nations have adopted AI significantly boosting their economic conditions.
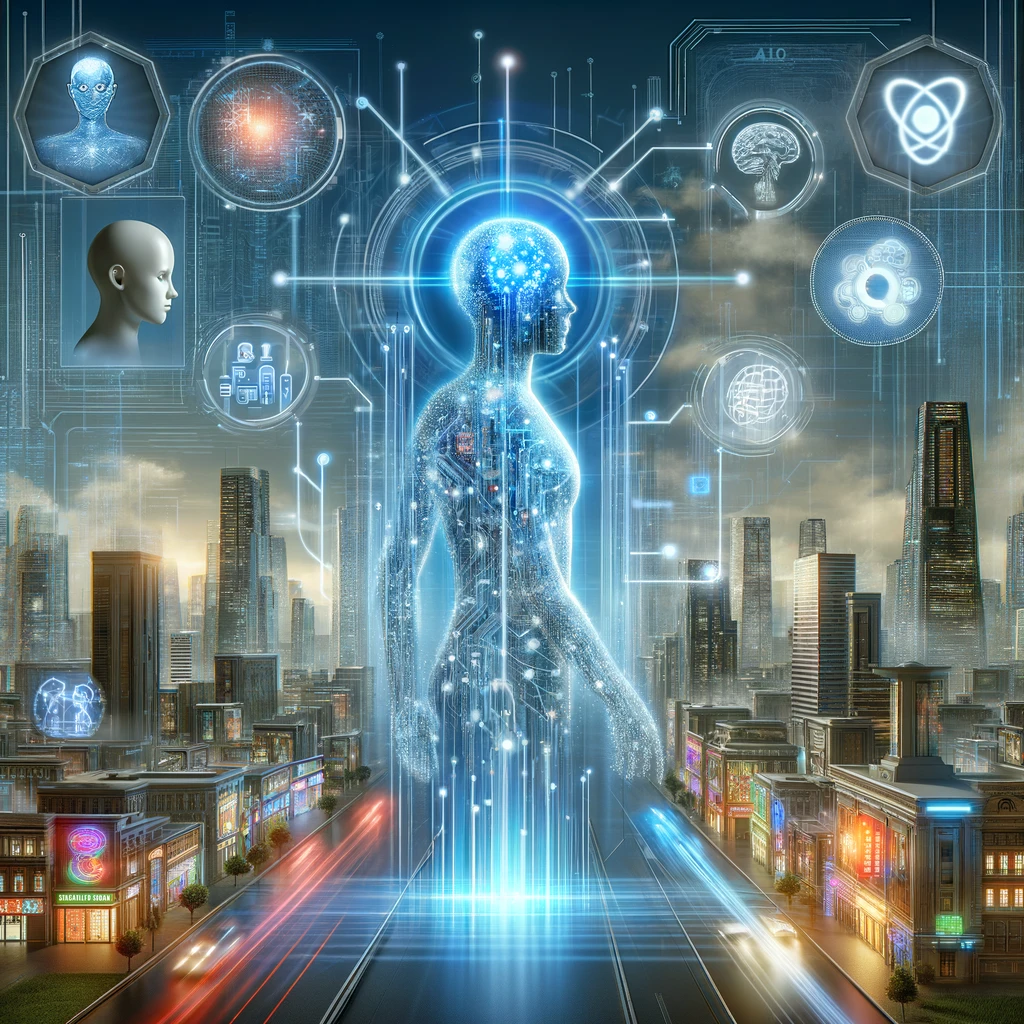
Understanding AI's importance in these nations is crucial due to their unique socio-economic challenges such as inadequate healthcare, infrastructure, and financial services. AI offers tailored solutions, boosts economic opportunities and enhances productivity. AI can also transform public healthcare improving patient experiences and outcomes and revolutionize finance through efficient trading and risk management. A study conducted by Temur Khabibullaev, Visa Inc., Hartwick College, Member, IEEE, Austin, Texas and Weian Wang, Computer and Information Sciences Department, Hartwick College, Oneonta, NY, aimed to explore the use and impacts of AI technologies, such as Generative AI, neural networks, and deep learning in various sectors of third-world countries. The primary objectives of this study were to investigate how AI addresses socio economic issues in third world countries and analyze how AI can generate revenue across various sectors in developing economies. Challenges and opportunities of using AI were also explored and various strategies were proposed for inclusive AI deployment and development in third world countries.
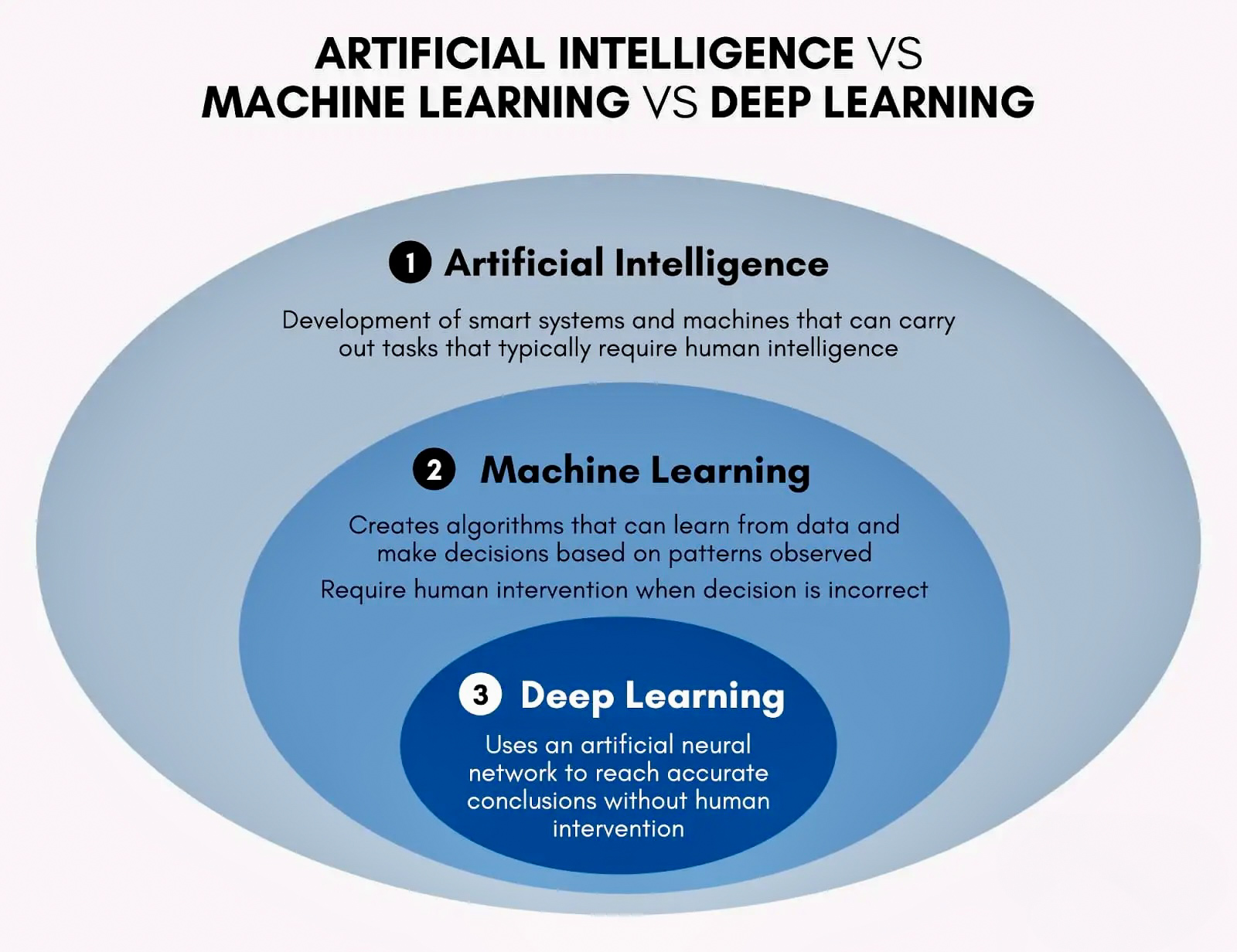
Technologies Explored in the Study
Generative AI: Generative AI represents a new type of machine learning that creates content similar to human-made work. It uses a variety of approaches through which images, text, music can be generated opening opportunities for creativity in art, culture, language translation and content creation. This can address resource limitations and enhance economic development in third-world countries by contributing to cultural enrichment.
Neural Networks: Neural networks simulate the human brain's functioning, offering solutions in image and speech recognition, natural language processing, and pattern recognition. Neural networks can solve a vast majority of socio economic challenges. This technology can be optimized for the development of urban infrastructure, to increase agricultural yields, and personalize learning.
Deep Learning: A subset of machine learning, deep learning involves neural networks with multiple layers. It excels in complex scenarios like visual identification and linguistic processing, addressing significant health, financial, and governance issues. Given the limited access to technology and knowledgebases in third-world countries, Deep Learning has the potential to significantly drive social and economic transformation.
Machine Learning: Machine learning encompasses techniques that allow models to learn from data without human intervention. It can boost agricultural productivity, enhance diagnostic methods, and improve healthcare facilities, promoting economic growth and better living standards.
Existing cases of AI in developing countries
Farming and Agriculture: AI tools have the ability to enhance productivity and manage natural resources which are vital for income and food security. Studies in East Africa and Pakistan show deep learning and AI-based systems improving water management, crop yields, and disease diagnosis. The report suggests that "small business owners may use their smartphones and low-cost sensors to monitor soil contamination levels, weather conditions, and crop health levels that are needed for AI-generated data and insights". Additionally, fog computing and AI prediction models help conserve resources and optimize farming practices.
Disaster prediction: AI models have the potential to predict and manage natural disasters effectively. For instance in Nepal deep learning models using Landsat images detect landslides with high accuracy. In Bhutan CNN-based models enhance landslide vulnerability mapping. AI also forecasts climate variability and flood risks as seen in Zimbabwe and Bangladesh improving disaster preparedness and response. Moreover the study also mentions that "in regions where there’s high risk of earthquakes, machine learning models can be used to accurately predict seismic performance, offering valuable insights for risk mitigation".
Economic forecasting: AI and machine learning improve economic predictions, aiding policy-making in developing countries. Studies in Bangladesh use the autoregressive integrated moving average model (ARIMA) for forecasting GDP, while neural networks along with support vector regression (SVR) models in African countries enhance GDP growth predictions. "The information on the economy that developing countries may derive from AI and machine learning allows them to comprehend their economic setting and assist them in determining where to put assets and how they can derive sustainable development" as mentioned in the study. Advanced computational techniques provide accurate economic insights, informing better investment and development strategies.
Healthcare: AI and machine learning improve healthcare outcomes by enhancing diagnostic accuracy and resource allocation in various countries around the world. In India for instance, hybrid models predict COVID-19 mortality rates with high accuracy. The study also suggests that "the application of artificial intelligence and machine learning in healthcare systems in developing countries presents a unique opportunity in significantly improving patient outcomes and tackling resource scarcity". In Pakistan for instance, ANN models forecast infant mortality trends, aiding health policy formulation. AI also supports healthcare information systems, improving user satisfaction and system adoption in hospitals.
These applications demonstrate AI's potential to address socio-economic challenges in developing countries driving growth, resilience and improved quality of life.
AI uses in finance in developing nations
Fintech and fourth industrial revolution technologies enhance financial inclusion and sustainable development in developing countries by increasing access to affordable funding. However, challenges such as bias perpetuation and increased risks accompany these benefits. In India for instance, the adoption of Generative AI (GenAI) in banking improves customer engagement and operational efficiency but necessitates strategic reskilling of banking professionals.
Barriers to AI adoption
According to the study, developing countries face significant hurdles in AI integration including inadequate high-quality datasets and poor technological infrastructure which hinder effective AI solution deployment in countries. Additionally a shortage of skilled professionals in AI, data science and machine learning further slows down the progress. Addressing these barriers requires concerted efforts from governments and the international community to create supportive legal, educational and infrastructural frameworks.
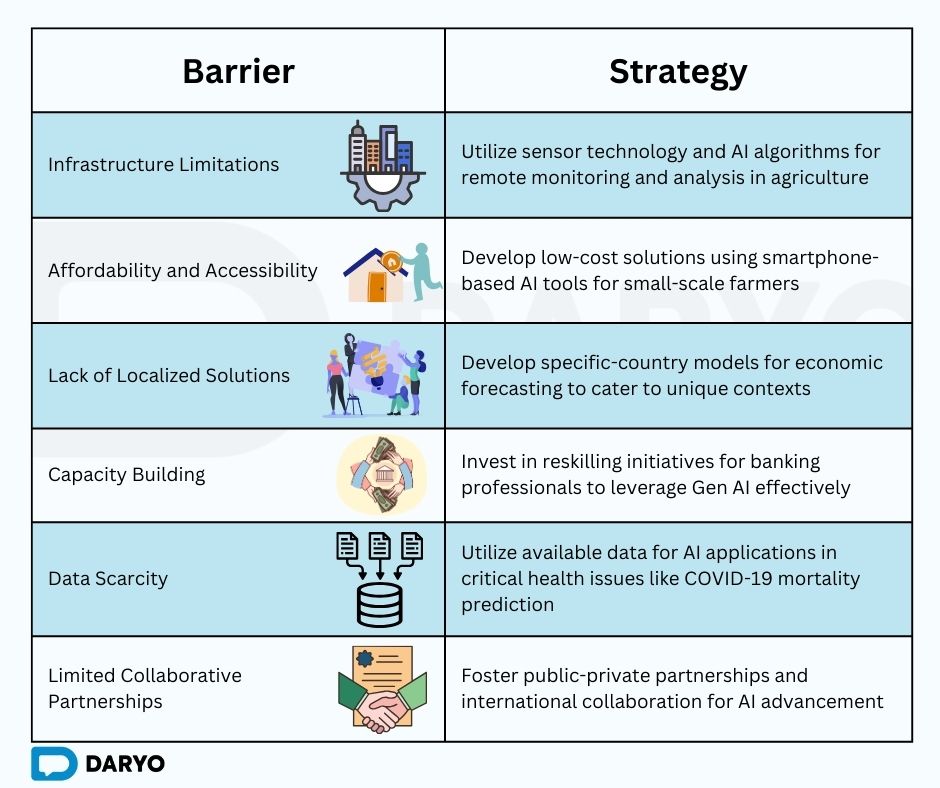
In conclusion, this research highlights the transformative potential of artificial intelligence in agriculture, disaster management, economic prediction, healthcare sector and finance in third-world countries. Generative AI, neural networks and deep learning show promising outcomes in the aforementioned sectors. However widespread adoption of AI today is hindered by limited access to quality data and inadequate infrastructure to support it. To overcome these barriers, governments of third world countries must collaborate with businesses and international agencies to promote scientific development and implement necessary reforms.
Comments (0)